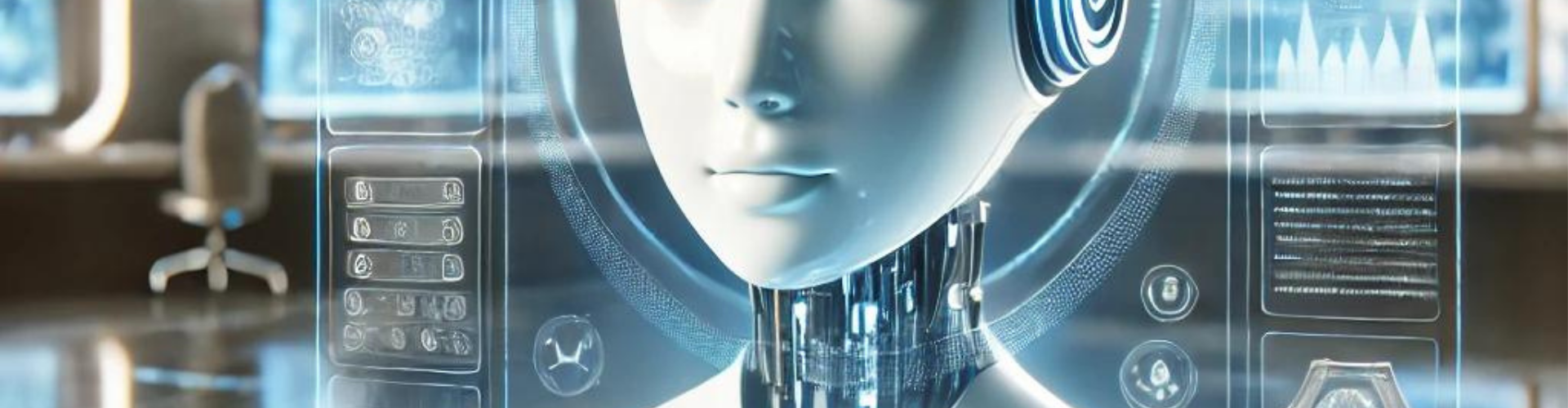
AI Chatbot Design
Crafting UI Copy and
Content Guardrails for
Amazon's A to Z Assistant
In response to a business need for streamlined access to information and HR administrative tasks, Amazon developed the A to Z Assistant (AZA), an AI-powered virtual assistant. AZA was designed to help Amazon employees find information and complete tasks more efficiently, freeing up valuable time for serving customers.
On average, Amazonians lose an estimated 30 million hours of productivity annually searching for basic information and navigating over 30 different tools. AZA addresses this by making it faster and easier for employees to get answers and complete tasks.
Employees interact with AZA using natural language queries. The Assistant leverages generative AI technology to provide answers from a vast array of content sources, both internal and external.
Specialised plugins enable automated workflows, allowing AZA to handle tasks such as booking time off, scheduling meetings, and creating tickets. Integrated into enterprise web and mobile search experiences, as well as internal tools like Slack, AZA offers context-specific answers and actions, available to employees whether they're in the office, at home, or on the move.
-
Hint text copy
When the user hovers over icons, hint text tells them what the elements enable them to do.
-
Beta tag copy
Since AZA was still in beta phase, we needed to find a way to manage users’ expectations. This copy informed the user that AZA was continuously learning.
-
Inactive state copy
After the user input a query, they are unable to do another input until AZA responds to their query.
Writing the chatbot
As the writer assigned to AZA, I crafted the UI and functional design copy. In addition, I conceptualised and implemented AZA's content guardrails. These guardrails were crucial for delivering valuable responses while mitigating risks associated with generative AI, especially when user prompts involved sensitive topics.
To ensure AZA provided reliable information, I defined content guardrails using specific mental models:
Internal Focus: AZA primarily helps Amazonians navigate internal policies and find information, enhancing efficiency.
Accuracy Over Guessing: AZA prioritizes giving no answer over a potentially incorrect one, preventing inaccuracies that could have serious consequences.
Conservative Blocking: Guardrails guide AZA in identifying and mitigating risks associated with sensitive topics, improving response quality.
I tested and refined these guardrails to control response behavior, ensuring AZA remained an effective tool. This involved setting custom guardrails to block specific phrases and topics, testing sensitive phrases, and documenting any inconsistent responses. By evaluating AZA's performance through customer feedback and response accuracy, I made iterative improvements to the guardrails.
AZA's predetermined response settings include:
"Sorry, I can't help with that." – For toxic content, blocked keywords, or sensitive topics.
"Sorry, I couldn't find relevant information to complete your request." – When relevant information is unavailable.
"Sorry, I'm having trouble fetching this response." – For technical difficulties.
Toxicity detection
To further ensure response accuracy and avoid inappropriate replies, AZA exclusively uses enterprise data from internal sources, excluding external world data.
To test and iterate the guardrails’ toxicity detection, I identified sensitive topics and phrases, prompting AZA with related questions. Documented responses are used as ground truth in daily accuracy tests, ensuring consistent and expected behaviour.
-
Examples of sensitive prompts and guardrails I
To test the toxicity detection of guardrails in AZA, I identified sensitive topics and words and asked AZA-related questions. Responses received are documented in the table. It’s the foundation from which I worked to design custom answers to toxic queries.
-
Examples of sensitive prompts and guardrails II
-
Examples of sensitive prompts and guardrails III